How do we come to know what we know?
Historically, this debate has hinged on the idea of innateness. Some thinkers, like John Locke, maintained that an individual is born a blank slate — a tabula rasa. Everything we come to know is learned exclusively via interacting with our environment. Others, like Noam Chomsky, argued that environmental stimuli are simply too impoverished to explain the vast richness of our linguistic prowess. It follows, then, that some knowledge must be innate.
At the Schwartz Reisman Institute’s Absolutely Interdisciplinary conference held in June 2023, Faculty of Arts & Science Department of Psychology professor and SRI Faculty Fellow William Cunningham and Google DeepMind Senior Research Scientist Joel Leibo illustrated an innovative method to perhaps settle this nature vs. nurture debate: reverse engineering our social behaviour through a piecemeal modelling of social cognitive theories using artificial intelligence (AI). Doing this potentially compresses a social phenomenon into its irreducible elements. Put another way, if we can program a computational model to exhibit a behaviour of interest, then we can possibly refute Chomsky’s innateness argument.
Cunningham and Leibo took turns describing studies that attempted to test the boundaries of this conditional statement. In doing so, they revealed how AI can not only refine our understanding of the building blocks of human behaviour, but also rewrite the rules of the scientific endeavour of social psychology itself.
A recipe for social artificial intelligence
Social cognition, the overarching phenomenon Cunningham and Leibo sought to model, refers to how people process, store, and apply information about other people in social situations. To do this, a human — or an artificial agent — must be able to learn from cues in their environment, transfer what they learned at one point in time to another point, and possess the appropriate neural architecture that allows for these processes to take place.
Using a deep reinforcement learning model satisfies these three requirements. First, its neural networks (like those found in a human brain) can learn patterns in and relationships between data and use this knowledge to make predictions. Second, it can use a recurrent neural network (a type of neural network that allows for the intertemporal transfer of what was learned) and contrastive predictive coding (a way of putting in prior knowledge or assumptions into a system) to enable artificial agents in the system to learn to “use their memories.” The result? An artificial agent that can interact, learn, remember, and predict — a great substrate for theory testing.
The origin question
Both Cunningham and Leibo spoke of the alluring simplicity of an assumption-free model in reinforcement learning. Traditionally, social psychology has worked by coming up with explanations for observed behaviour. These explanations are assumed to exist a priori. But can we still get a given phenomenon to emerge even when we don’t hardcode these a priori assumptions into our model?
According to their multi-agent reinforcement learning study on ingroup bias, we can. Social psychologists have tended to chalk up peoples’ preference for ingroups and avoidance of outgroups to core human motivations. Perhaps we have a coalitionary instinct, or a need to belong, or a need for group dominance which explains this bias. What makes reinforcement agents great for testing this theory, Cunningham asserts, is that they have none of these. So, finding that ingroup bias still emerges in a simple agent-reward environment possibly refutes the innateness of these explanations.
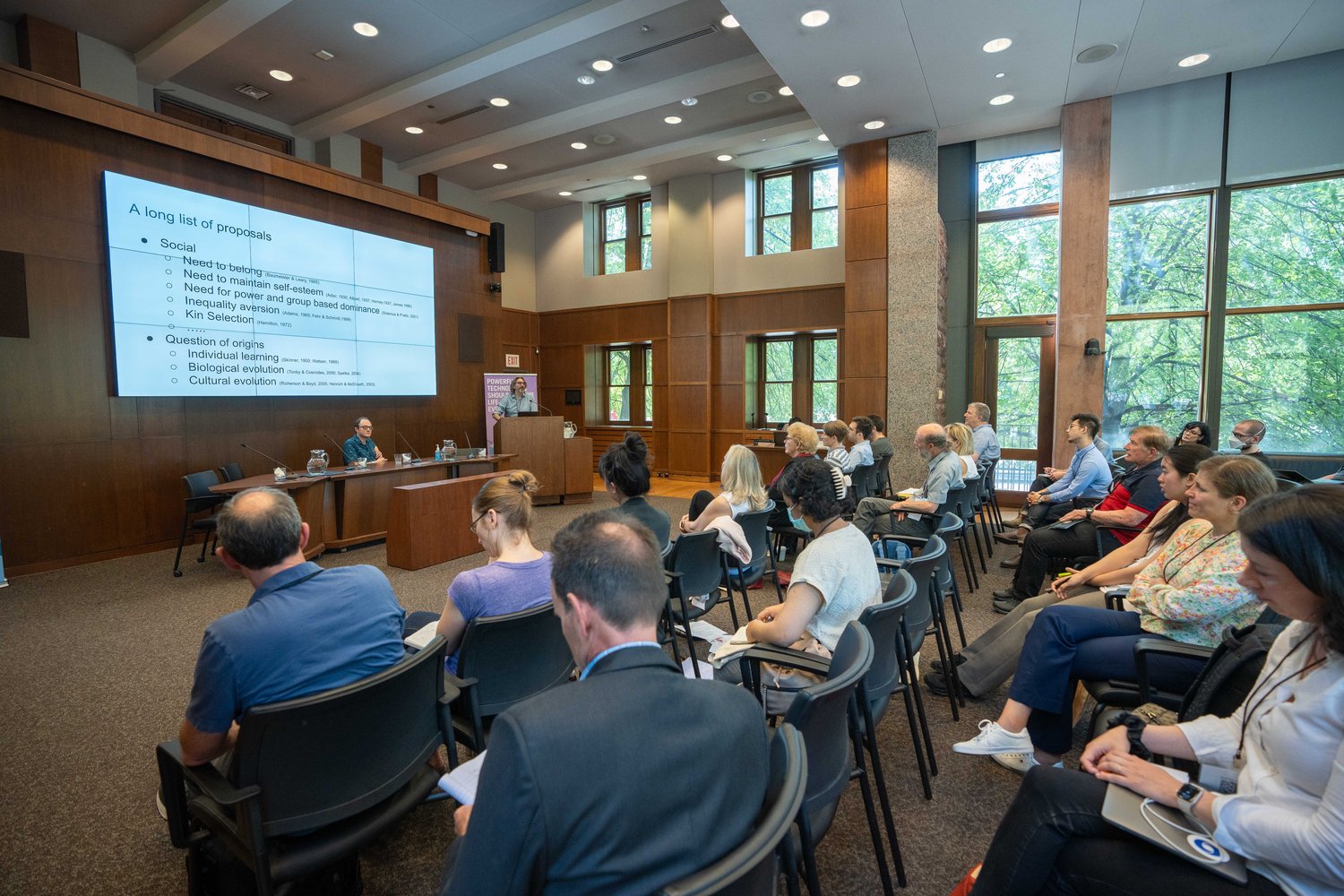
Us vs. them and the luxury of assumption-free models
To test this, Cunningham and Leibo created an artificial environment where “Red” agents were trained to first collect differently-coloured resources available in the environment. Then, for the reward, each agent interacted with the other agents as a function of how closely the colours of their respective resources matched. Meanwhile, in a separate environment, “Blue” agents were busy doing the same. Every so often, both Red and Blue agents would get dropped into a mixed environment where they could interact with agents from the other colour group based on the same rules.
Cunningham interestingly notes that neither the Red nor Blue agents were explicitly told to only interact with agents from their colour group. The reward that these agents were seeking to maximize (i.e. the colour coordination of resources) is not connected to the colour of the agent to whom the resources belong. In other words, none of the agents were programmed to be biased in favour of their ingroup.
Yet when both Red and Blue agents were later placed in a testing environment together, Red agents interacted more with other Red agents and Blue agents interacted more with other Blue agents. And this effect persisted even when all the resources on which the agents would coordinate — the source of the reward — were green. If all resources are green, there becomes no real difference in the actual rewards the agents are receiving. The only difference would be the amount of time and exposure the agents had had with each other prior to testing. This was the source of the group-based bias.
Here, Cunningham used AI models to illustrate and refine the origins of the social phenomenon of ingroup bias using no prior assumptions — something traditional social psychology methods have thus far been unable to do.
The unexpected virtues of envy
As in the case of the Red and Blue agents, sometimes all you need to specify for a behaviour to emerge is the reward. Learning can figure out the rest. Other times, this set-up causes the system to collapse on itself.
In a multi-agent “tragedy of the commons”-style study, Leibo and his colleagues demonstrated a scenario in which agents maximizing a reward in the short term counterintuitively blocks long-term reward maximization. In these cases, adding in prior assumptions like intrinsic motivations becomes necessary to achieve a viable system.
Leibo’s rules were simple. Agents are rewarded when they eat an apple. Apples only grow if the patch they are in is sufficiently dense. Patches maintain the required density only if given enough time to re-grow their apples. Agents can also zap other agents to temporarily exclude them from the environment – but this is only a secondary feature of the environment that is not explicitly related to the apple eating. All in all, if the agents eat too many apples too fast, the apples don’t grow back, and everybody starves.
When there is just one agent in the environment, it is able to learn not to overconsume because it is able to experiment. It tries eating less and observes the positive outcome of non-starvation that results. However, as Leibo explains, in a multi-agent version of the environment, there is no way for an individual to perform that experiment. If one agent tries eating less, another agent is likely to eat more. The association between eating less and non-starvation is broken. The result is that all agents learn to eat as fast as they can in hopes of grabbing one extra apple ahead of others, so they end up rapidly depleting their environment and starving.
The solution? Adding “disadvantageous inequity aversion” into the agents — or envy, as Leibo more informally calls it. To do this, Leibo and his colleagues programmed each agent to, in addition to eating apples, monitor how much lower its individual reward is compared to every other agent. These differences get summed and the aggregate becomes a penalty (i.e. a deduction) on the agent’s real reward. This motivates the agents to decrease this penalty. Over time, they learn to do this by zapping other agents that are eating too much too quickly. In turn, the zapped agents learn that eating too much too quickly now leads to temporary exclusion from the environment. The overall effect is that the agents, by virtue of this prior assumption of envy, now police their own and each other’s behaviour, ensuring that the apples stick around for a longer period of time.
Leibo’s study demonstrates the benefit of a bottom-up, piecemeal building of social cognitive models enabled by AI. By figuring out the “next step up” from total collapse, we can use these models to define and test the true building blocks of social behaviour.
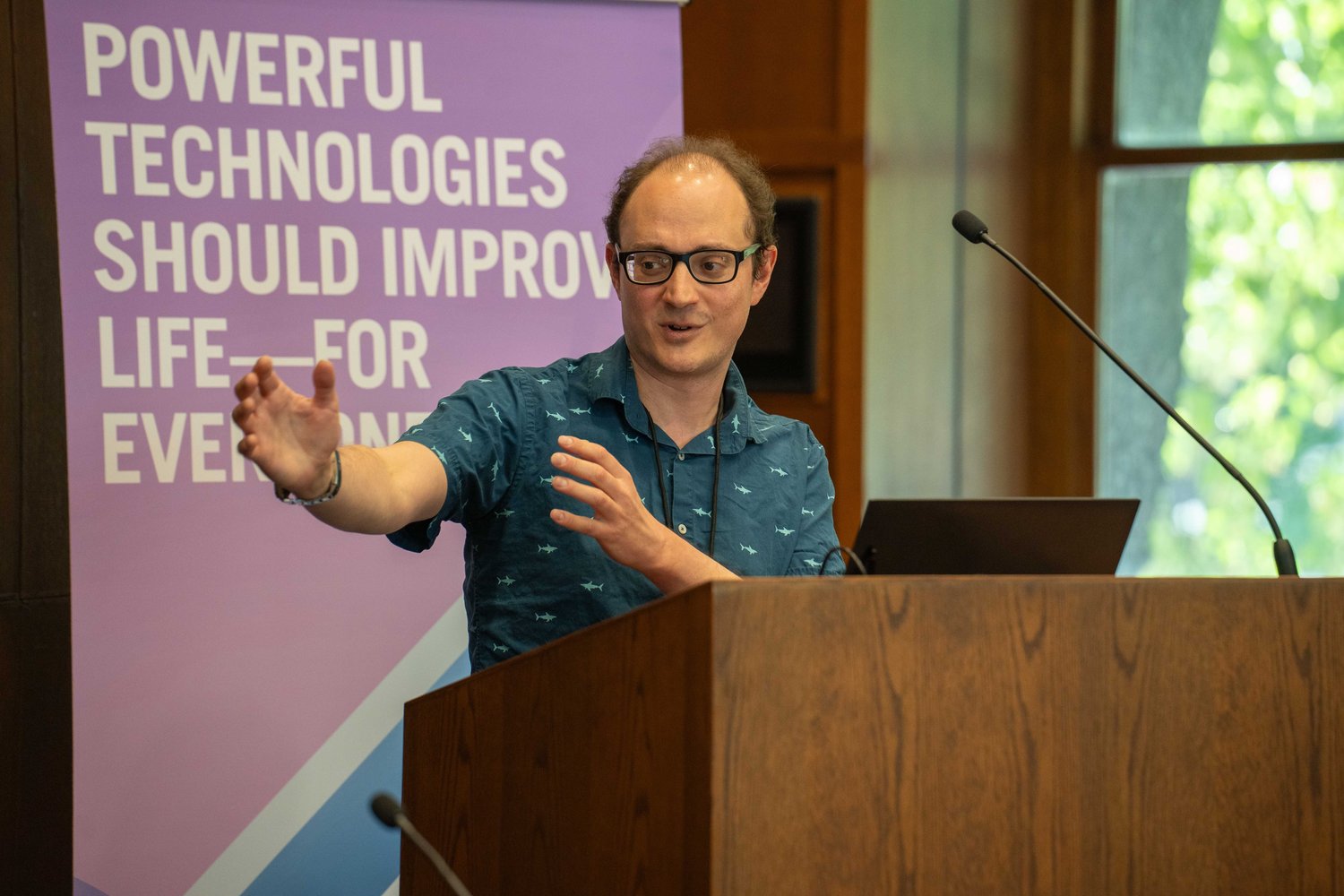
Rewriting the rules
What do these findings mean for the scientific endeavour of social psychology?
Cunningham notes that these AI methods could allow social psychologists to test their proposed solutions — whether social, political, environmental, or otherwise — by scaling them in a low-stakes artificial environment first. This would save all stakeholders time, effort, and money, while also avoiding the use of humans as guinea pigs for potentially unethical interventions.
More existentially, these methods have the potential to redefine how we think about the study of human behaviour in the first place. For example, most attempts at theory testing in traditional social psychology have been top-down approaches. We observe a behaviour of interest and work our way down hypothesizing its possible causes. But what if we flipped this process on its head and worked upwards, adding and tweaking until we find, as Leibo put it, the “minimal set of maximally general priors?”
Watch the session recording:
Reem Ayad is an SRI Graduate Fellow.